1. 理論
2. 学習
2.1. 基本
モデルを宣言し、fitでデータを当てはめます。
当てはめた後のオブジェクト(gmm
)が学習後のモデルです。
means_
メソッドやbic
メソッド、predict
を利用して下流の解析に用います。
# libraries from sklearn import mixture from sklearn.mixture import GaussianMixture # data xy = np.array(res_decomposition.iloc[:, :2]) # fit gmm = mixture.GaussianMixture( n_components = 50, covariance_type = 'full', # ['spherical', 'tied', 'diag', 'full'] n_init = 10, reg_covar = 0.1, random_state=42 ) gmm.fit(xy)
2.2. BICによるモデル選択
ハイパーパラメータをBICに基づいて選択し、ベストなモデルを返す関数とその実行例は以下です。
def calc_gmm_BICs( res_decomposition, n_component, cv_types = ['spherical', 'tied', 'diag', 'full'], reg_covar = 0.1 ): # to nparray xy = np.array(res_decomposition.iloc[:, :2]) # BIC BICs = [] best_gmm = None lowest_bic = np.infty lowest_bic_cv_type = "" lowest_bic_n_component = 0 n_components_range = range(1, n_component + 1) n_init = 10 for cv_type in cv_types: for n_components in n_components_range: gmm = mixture.GaussianMixture( n_components = n_components, covariance_type = cv_type, n_init = n_init, reg_covar = reg_covar, random_state=42 ) gmm.fit(xy) BICs.append(gmm.bic(xy)) if BICs[-1] < lowest_bic: lowest_bic = BICs[-1] best_gmm = gmm lowest_bic_cv_type = cv_type lowest_bic_n_component = n_components return { 'best_gmm': best_gmm, 'BICs': BICs, 'lowest_bic': lowest_bic, 'lowest_bic_cv_type': lowest_bic_cv_type, 'lowest_bic_n_component': lowest_bic_n_component, 'n_component': n_component, 'cv_types': cv_types, 'reg_covar': reg_covar } # PCAとUMAPの各々についてBICを計算 bic_results_pca = calc_gmm_BICs(res_pca, 50) bic_results_umap = calc_gmm_BICs(res_umap, 50) # 結果の保存 no = now() os.makedirs(f'{TEMP_DIR}/best_gmm/{no}') pickle_save(f'{TEMP_DIR}/best_gmm/{no}/bic_dict_pca.pkl', bic_results_pca) pickle_save(f'{TEMP_DIR}/best_gmm/{no}/bic_dict_umap.pkl', bic_results_umap)
上記の結果を棒グラフで可視化する関数は以下です。
# library import itertools # Plot the BIC scores def plot_gmm_BICs(bic_results, cv_colors = ['blue', 'green', 'red', 'orange']): # unpacking BICs = np.array(bic_results['BICs']) n_components_range = range(1, bic_results['n_component'] + 1) cv_types = bic_results['cv_types'] # figure plt.figure(figsize=(14, 6),dpi=100) ax = plt.subplot(111) # bar plot bars = [] cv_colors_iter = itertools.cycle(cv_colors) for i, (cv_type, color) in enumerate(zip(cv_types, cv_colors_iter)): xposes = np.array(n_components_range) + .2 * (i - 2) bars.append(plt.bar(xposes, BICs[i * len(n_components_range):(i + 1) * len(n_components_range)], width=.2, color=color)) # text (place * near the best model) xpos = BICs.argmin() % len(n_components_range) + .65 + .2 * BICs.argmin() // len(n_components_range) plt.text(xpos, BICs.min() * 0.97 + .03 * BICs.max(), '*', fontsize=14) # layouts plt.title(f'BIC score per model') plt.xticks(n_components_range) ax.set_xlabel('Number of components') plt.ylim([BICs.min() * 1.01 - .01 * BICs.max(), BICs.max()]) ax.legend([b[0] for b in bars], cv_types) # shoe plt.show()
上記関数の実施例は以下です。
plot_gmm_BICs(bic_results_pca)
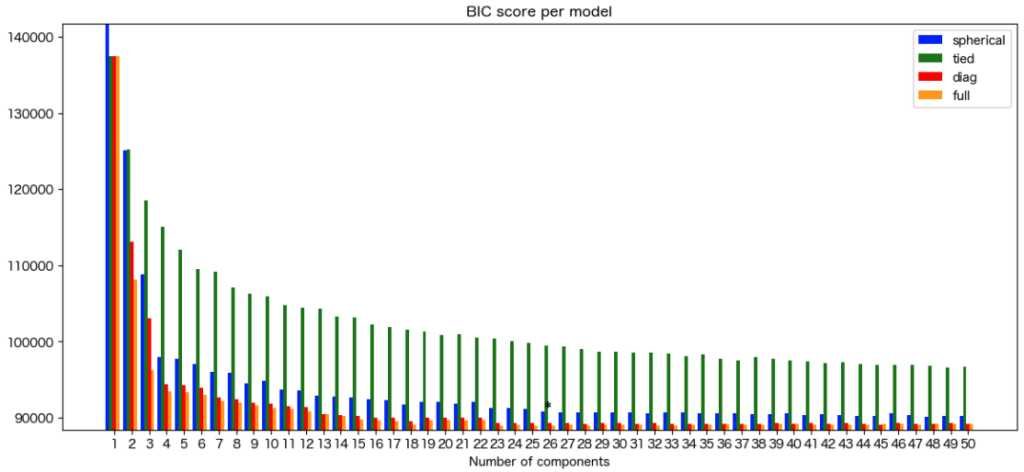
3. 平均・分散・AIC・BIC
# 平均 (各componentの平均) mean_x = best_gmm.means_[:, 0] mean_y = best_gmm.means_[:, 1] # BIC gmm.bic(xy)
4. 予測
どのcomponentに属するか予測(クラスタリング)
clusters = [i + 1 for i in best_gmm.predict(xy)]
5. 等高線による可視化
関数と実行例は以下です。
def plot_decomposition_with_best_gmm(data, bic_results): # to nparray xy = np.array(data.iloc[:, :2]) # unpacking best_gmm = bic_results['best_gmm'] lowest_bic_n_component = bic_results['lowest_bic_n_component'] lowest_bic_cv_type = bic_results['lowest_bic_cv_type'] # plot decomsition plt.figure(figsize=(14, 10)) plt.scatter(xy[:, 0], xy[:, 1], s=5, label='Data', color='blue', alpha=0.5) # plot cluster centers plt.scatter(best_gmm.means_[:, 0], best_gmm.means_[:, 1], marker='o', s=100, label='Means', color='red', edgecolors='black') # plot contours x_min, x_max = min(xy[:,0]), max(xy[:,0]) y_min, y_max = min(xy[:,1]), max(xy[:,1]) x_delta, y_delta = x_max - x_min, y_max - y_min x = np.linspace(x_min - 0.05 * x_delta, x_max + 0.05 * x_delta, 100) y = np.linspace(y_min - 0.05 * y_delta, y_max + 0.05 * y_delta, 100) X_grid, Y_grid = np.meshgrid(x, y) Z = -best_gmm.score_samples(np.array([X_grid.ravel(), Y_grid.ravel()]).T) Z = Z.reshape(X_grid.shape) plt.contour(X_grid, Y_grid, Z, levels=10, linewidths=1, colors='green', linestyles='dashed', alpha=0.5) # layout plt.xlabel('Axis 1') plt.ylabel('Axis 2') plt.title(f'Gaussian Mixture Model with {lowest_bic_n_component} components and {lowest_bic_cv_type}') plt.legend() plt.grid(True) plt.show() plot_decomposition_with_best_gmm(res_pca, bic_results_pca)
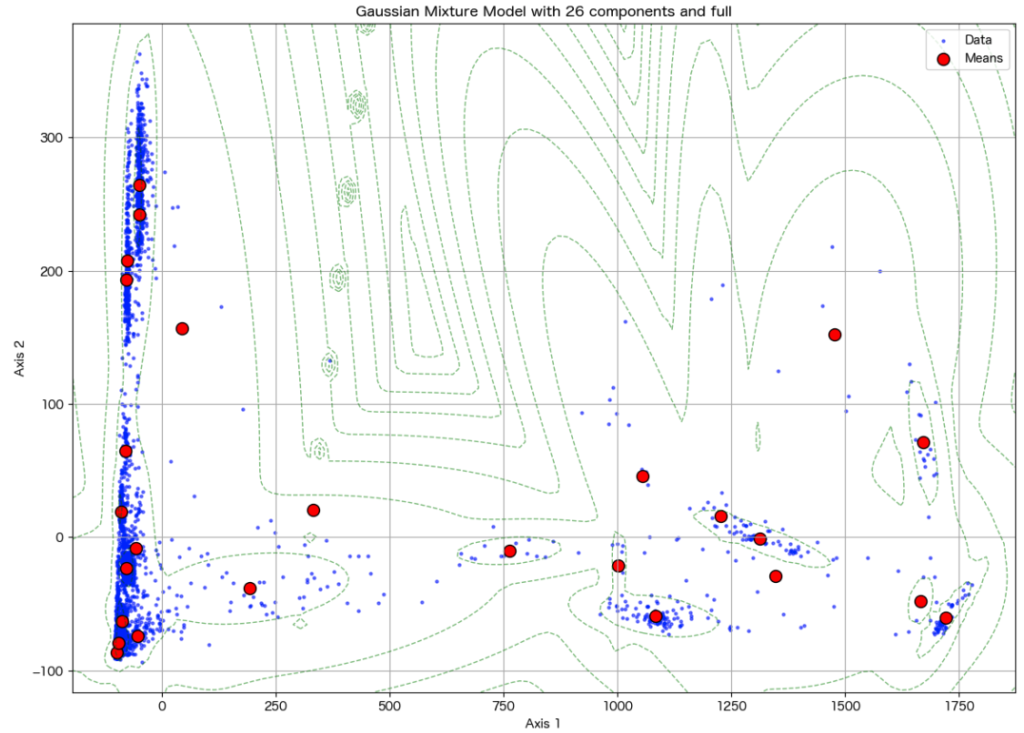
コメント